Decision science draws on both mathematical models and human intuition in offering a formal framework for making decisions. The tools can aid in a range of areas, from testing academic theories to assisting individuals making life-and-death decisions about medical treatments. Global Network Perspectives talked about the field with Itzhak Gilboa, the AXA Chair for Decision Sciences at HEC Paris.
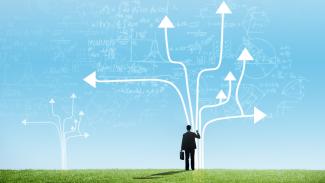
What does decision science do?
Decision science has two core questions: how do people make decisions (“descriptively”), and how should people make decisions (“normatively”). Both are important.
The emphasis would change depending on who you are working with. An economist will often be more interested in the descriptive side, say, trying to predict agents’ behavior in order to feed it into a model that analyzes how markets would respond to a change in interest rates. In this setup you’re interested in how people behave, not how you think they should behave. By contrast, talking to business school students the normative side is much stronger: you talk about how those future leaders should make decisions.
What is this “should”? How do I know? When I’m teaching decision theory I don't want to say, “I am the professor, therefore I know the answer.” I want to help people with the process of making decisions. I can provide formal structures that can do so, while they still let people make their own decisions.
Take something very simple as an example, the principle of transitivity—if you prefer A to B, and B to C, you should prefer A to C. If I can show students situations where, in this setup, they actually choose C over A, I try to see if they feel comfortable with these cyclical preferences. And if they don’t, then I can also give them some tools to make sure they avoid committing the same “mistakes” in the future.
Another simple example is the framing effect. If I have to decide whether or not I should undergo a certain operation and I’m told there’s 30% probability of death, that sounds bad. If I’m told there’s a 70% probability of survival, that sounds good. It’s the same operation, the same outcomes, but depending on how it’s framed, we respond differently. When people feel uneasy about being sensitive to the “frame” I can give them a tool that helps: using formal models. Putting the decision in a model won't give me the “right” answer; I still have to make a decision that’s right for me. But just writing it down in a formal model would make me immune to the framing effect.
I start a course teaching decision theory with tenets that are more or less universal, but gradually I’m presenting axioms and principles over which views tend to differ. I’m trying to convey the idea that if it's your decision, you will also have to decide which principles to obey.
How is decision theory applicable?
I think that decision theoretic models can be applied and can be useful in a variety of ways. On one end of the spectrum there is the Google Maps example, where all of the data is there. The algorithms give you the answer, and that's the end of the story, no ifs, ands, or buts. You want to get from point A to point B in the city. Your intuition tells you to turn right; Google Maps says turn left. Turn left. The problem is fully analyzed and you shouldn’t argue with the math.
On the other end of the spectrum, there decisions in which models can’t help at all. There are very few decisions where I can say that intuition is the be-all and end-all. Maybe getting married is the only example I can think of.
Most important decisions in our lives are in between these extremes. We don’t have models and data that render intuition useless. But we also don’t want to ignore the models. It's wonderful you have intuition. I don't want to erase it. I need it for my models, because otherwise my models are just symbols. But let's try to work together to see whether I can formalize it, to see how robust it is. Sometimes this very dialog will change your mind. Sometimes because of the very fact that I ask you questions, you find yourself giving different answers.
When it comes to what to do with your life savings, we don't have the information for a comprehensive answer that the computer will calculate for you, as in the case of Google Maps. But you can talk to a decision theorist before you make a final choice. Suppose I come and sit with you and ask, “Let's try to rationalize your decision, post hoc. What are your goals? What are your beliefs? What hidden, implicit beliefs might be playing in? Before you push the button, let's try to see what assumptions we need to make in order to justify your decision.”
One lesson we should be trying to get across to business leaders is that people tend to think of complex decisions as either/ors. Either there is a mathematical algorithm that lets the computer tell me what to do, or it's hopelessly difficult and I'll just follow my intuition. But, while most important decisions in life are such that we don't have the models or the data that would give you the right answer, there is a whole spectrum of ways in which decision theory can be useful. Importantly, some of them require learning the language to have a dialog with decision theorists.
Where did decision science come from?
Decision theory is a way to think about the world. There is an elegant and amazingly deep body of theory on rational choice. We have constrained optimization, decision making under uncertainty, expected utility, game theory, Nash equilibrium and refinements thereof, the basics of microeconomics, and some social choice.
I would say that this paradigm is one of the greatest achievements of the 20th century. I wouldn't put it on par with quantum physics, but it's still one of the big intellectual achievement of this past century. Some of the principles go back 350 years, but there are quite amazing contributions made by von Neumann and Morgenstern, Arrow, Nash, Savage, and other luminaries.
When you go back, you see that many of these ideas resided back in the writings of mathematicians and philosophers like Pascal, Bernoulli, Leibniz, and Descartes. But economics and operations research gave it a big push. Much of its development happened in tandem with economics in the 1930s and 1940s when the applicability of these ideas was demonstrated. Beyond that it was helped by other social sciences where decision theory was seen as a useful way to model phenomena.
What’s next for decision science?
The field is at a very important and interesting time because this tremendously beautiful paradigm is confronted with evidence from psychology—mostly by Kahneman and Tversky and their followers—showing that people don’t always behave according to the theory. This obviously raises a challenge to the theory as a descriptive one. But in some cases, the normative appeal of the theory is also questioned, where people do not obey its dicta but also refuse to change their behavior even after the theory has been explained to them.
This conflict implies that there are choices to be made. First, between the descriptive and the normative: in face of this discrepancies, are we going to fix the theory so that it would be a better description of reality, or go out and preach it, normatively, so that reality would come closer to the theory, as it were? In any event, we have to accept that we can’t always use the same theory as an acceptable normative standard that will simultaneously describe how people actually make choices.
Second, I think the discipline is at a point of further bifurcations even within the two main applications. We have probably exhausted the broadly applicable common ground. It's a process that game theory has already undergone, so that may be a useful analogy. Early on, just using the idea of a game—strategies, players, payoffs, beliefs, equilibrium—was wonderful. It can be a model of any kind of interaction—between you and your neighbor, between two countries, between buyers and sellers, between animal species, or between computers trading on markets. It's amazing.
Part of the beauty is that you have something that's common to all of these things. That in itself is really an eye-opener, and it allows you to see analogies. To see such analogies without the formal model takes a brilliant mind. But, when you describe situations and write them down as games, analogies become quite clear. Suddenly you can see that structure everywhere. It is fantastic—up to a point. But eventually, what you can say about this common ground is exhausted.
These days work in game theory is growing increasingly specific. More often than in the past, a model will apply only to auctions or only to biology. You cannot always have the same answer to all of these categories.
I think that decision theory is already undergoing a similar process. We’ve had expected utility maximization as the foundation, and it was beautiful and insightful for almost any decision we could think about. But when we try to move forward and cope with the challenges posed by psychology, we probably cannot expect one model that does it all.
Where is decision science used currently?
The two main markets where decision theory is selling its products are academics using it in their modeling and people who are actually making decisions.
A friend and colleague, Peter Wakker at Eramus University in the Netherlands, worked for many years on medical decision making. He worked with people and advised patients about whether to undergo an operation or not. This is an application that’s very different from applications to economics. Deciding whether to amputate a leg or not is a completely different kind of question than, say, the theoretical impact of taxes.
Your preferences for the model at some point changes. An academic economist can do more things in the model if it is general, and has many degrees of freedom. But if you are actually talking to a person who is sick and making a very difficult decision, you don't want too many parameters. That person would value something precise. The same bifurcation would be true for social scientists needing to understand a political phenomenon and a person who needs to invest their money.
Is big data and increasing computational power impacting decision science?
If you were to talk about applications to concrete decisions like medical decision making, definitely.
If we’re talking about producing models for the social sciences, then the answer is, not so much. Scientists often want models that will help them test a theoretical argument. The actual data are usually not good enough for them. What they are doing is sometimes more in the realm of reasoning and argument.
What is the dynamic between theory and real world experience?
Amos Tversky used to say, “Give me the axiom; I'll design the experiments to refute it.” And this was not sheer boasting. He delivered. So the question is, what do you do when you have this conflict where the theory and reality are not aligned? You could bring the theory closer to reality, or reality closer to the theory.
Economic theory is supposed to be a description of how people actually make decisions. If people like Tversky show me that's not how they make decisions, one way I could deal with this is to say, let's change economic theory, and that's basically what behavioral economics has been trying to do.
The other direction could be maybe we should teach people how to make better decisions. So for instance, Kahneman and Tversky found that people confused the probability of A given B with B given A. People just mix it up. That is a very solid finding. Here in Paris they had an ad for the national lottery that said, “One hundred percent of the people who won the lottery bought a ticket.” It's funny. But it's also a very cynical exploitation of these psychological biases.
A less cynical approach might be to say, “Wait a minute, maybe the right thing to do is to teach this so people aren’t susceptible to it.” That can be pretty powerful.